This article is the second of a three-part series on optimizing airline ancillary bundles based on customer preferences. Part one discussed data collection and customer segmentation methods. In this section, we will cover recommendation and offer engines.
Caroline is looking for a flight to Barcelona from her home in London. It’s a two-year anniversary gift for her boyfriend Mark with whom she wants to spend a romantic weekend in Spain. On the airline website, Caroline is presented with three offers: the first one is basic, with just carry-on luggage and no seat reservation; the second one includes a snack and a beverage, a reserved seat with more legroom and priority boarding; the third one adds a 23kg hold bag and free rebooking. Caroline goes for the second option. It’s ideal for the couple – they want a hassle-free, enjoyable experience, but are planning to travel light anyway.
Caroline is happy with her purchase and her experience. But how did we get there? What happens in the background? How did the airline deliver an offer to Caroline that she enjoys and wants to book?
Recommendation Engines
The first step in getting the right offer to Caroline is the proposed trip purpose segmentation discussed in the previous article. The information gained here can be enhanced through a process called market basket analysis that helps the retailer recommend relevant content to offer. Essentially, this is a statistical analysis process that identifies purchasing patterns by identifying the relationships between items customers frequently buy. For instance, a review of the airline’s historical data might reveal that a customer who selects priority boarding also prefers a front-row seat. This correlation can be used to obtain item preference lists (a list of items ranked from most to least often purchased) for a certain customer segment. This helps the airline create relevant offers, even if the customer has not been identified (i.e. anonymous shopping).
The next level of offer customization is one-to-one personalization – this is possible if the customer has been identified as this allows the retailer to take into consideration prior sales history, stated profiles and natural language processing of user-review data for sentiment analysis to help recommend relevant products.
The personalized offers that are presented to Caroline are obtained by combining the aggregate trip-purpose segment and individual customer history using a weighted average. The weight to apply to each data source is based on the available customer-specific history by trip-purpose segment – the richer and more relevant the history for a customer, the greater the weight of her historical preferences. So, if Caroline is a frequent customer of the airline with well-established preferences, this history is weighted heavily; if she rarely books with the carrier, the market basket analysis will take precedence. At Sabre, this process of combining preferences for personalization is known as the blending algorithm.
Offer Engine
Unfortunately, we can never know with certainty a customer’s exact preference or willingness to pay. We can make an educated guess using information on historical preferences by trip-purpose segment, stated preferences in the customer profile, recent purchase history and user-reviews. Nonetheless, we should allow for the possibility that our estimated trip purpose segmentation classification and/or the preference ordering for this customer is incorrect. For instance, Caroline might be frequently traveling to Barcelona to visit a customer for the business which might mean that the airline has rich historical data on her preferences. However, Caroline’s requirements might be different when she’s planning a romantic weekend getaway with her boyfriend.
To account for the risk of segmentation inaccuracies, we typically recommend presenting multiple, diverse offers to improve the likelihood of finding a good match with the customer’s needs. Our practical recommendation is that, in addition to providing a “best fit” bundle solution (from our recommendation engine), we also include a separate bundle offer (“popular picks”) based on items preferences that are not trip-purpose specific (i.e. they are based on a simple, overall item popularity rank). The rationale for this second bundle is that, if trip purpose analysis doesn’t lead to a good match with the customer’s preferences, then overall item popularity should be a reasonable recourse. Based on her history with the airline as a weekday business traveler, Caroline might be provided with an offer that ideal for her needs in that context; however, when she’s traveling with her boyfriend, a more generic offer based on data from weekend leisure travelers might be the better fit.
In addition to “popular picks”, adding a third “inferior” version of the “best fit” bundle is another consideration. It’s a widely used marketing concept in which a closely similar variation (but deliberately worse in both price and content) of the “best fit” offer is presented to the customer. The idea is that people tend to evaluate items on a relative rather than an absolute basis; comparing a similar (but inferior) alternative generates a cognitive bias towards the preferred one and improves conversion rates[1].
One of the most important functions of the offer engine is the pricing decision. In Sabre’s experience, a useful approach for pricing offers is dynamic discounting – the higher the revenue of the bundle, the greater the discount (versus the full price of the individual items). A simple model for applying such progressively increasing discounts with revenue spend results in a concave curve such as shown below – non-discounted total price in shown on the horizontal axis (with average spend normalized to a value of 1), and the applicable discount percentage is shown on the vertical axis. This approach is widely used in many industries, is simple to manage and is widely accepted by consumers.
In part three, we will cover the use of experimentation engines to provide a “test-and-learn” framework to further improve our marketing effectiveness for ancillary bundles.
[1] An excellent depiction of these asymmetric dominance effects can be found in Dan Ariely’s book, Predictably Irrational (2010, Harper Press).
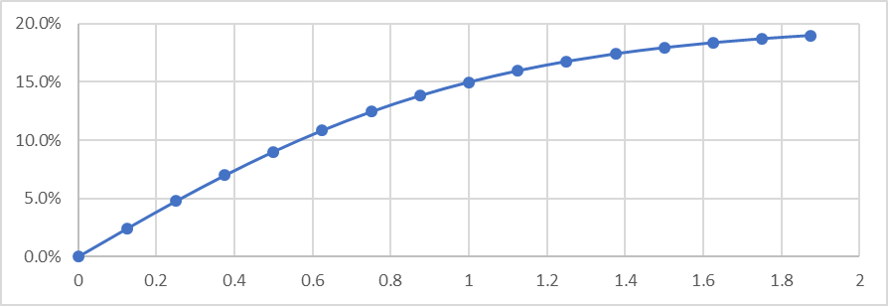